Anomaly Detection
Anomaly detection is a vital data analysis task where algorithms identify outliers or deviations from expected patterns, signaling potential issues. Detecting anomalies prevents data misrepresentation and addresses underlying problems. Effective anomaly detection delivers business value, including cost reduction, customer retention, and optimized resource allocation. Machine learning (ML) techniques enhance anomaly detection by improving speed and accuracy, enabling timely insights.
In machine learning, anomaly detection applies models to quickly identify anomalies in complex datasets. This ensures data integrity, which is crucial for reliable analysis and informed decision-making. High-quality data allows businesses to derive accurate insights, leading to better strategies.
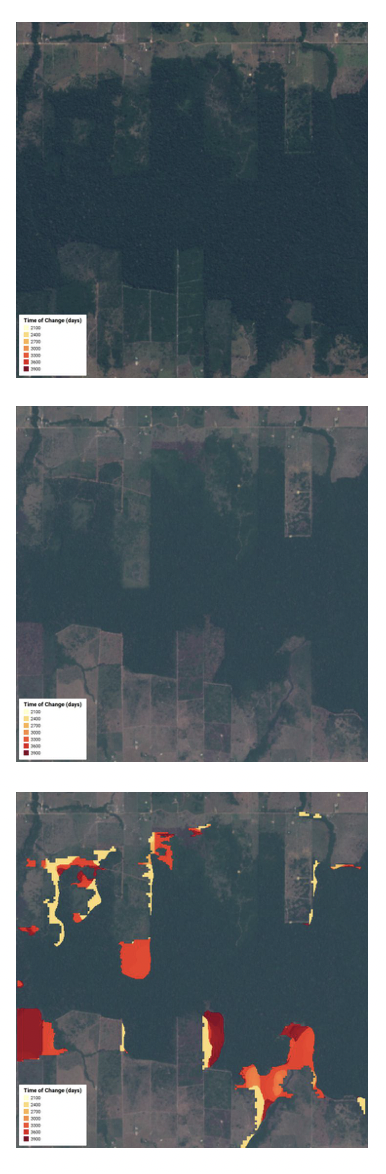
Deforestation
Deforestation has been identified as a vari- able of greater impact than climate change alone, as the single most important factor affecting ecological systems, and great- est threat to biodiversity. Due to availability of massive remote sensing satellite datasets, deforestation in critical forests such as the Amazon is now possible to detect. However, tracking of deforestation, degradation and regrowth of forests is a major challenge due to interference from transients such as clouds and shadows. Our team is developing a novel direction for such detection, framed in a new mathematical con- text. This involves functional analysis of tensor product rep- resentations and corresponding multilevel spaces, based on the Karhunen-Lo`eve (KL) expansion in functional data analysis. In contrast to cur-
rent statistical approaches, with its foundation
in functional analysis of tensor product expan-
sions, the proposed approach has many useful
properties well suited for detecting and track-
ing hidden phenomena on complex domains.
CONTACT
Stochastic Machine Learning Group
- Department of Mathematics and Statistics Boston University, 665 Commonwealth Ave. Boston, MA 02215
- + (617) 353-9549
- jcandas@bu.edu
- mkon@math.bu.edu
QUICK LINKS
Privacy and Terms
© 2024 – 2025, Stochastic Machine Learning Group